IHME, Microsoft, and Planet Collaborate to Map Climate-Vulnerable Populations In Unprecedented Detail
From intensifying natural disasters and food insecurity, to public health crises and forced migration, we live at a time of unprecedented climate risks. Each of us is exposed to these risks in a different way, and we do not all bear them equally. Yet no matter which risks we face, one thing is certain: on a warming planet, knowing where people are is critical to saving lives.
In an age of seemingly ubiquitous realtime information, it’s perhaps surprising that many of the world’s most climate-vulnerable populations simply aren’t on any map. They are excluded largely as a consequence of unplanned growth, economic and climate precarity, and migration.
Across the Global South for instance, many cities and regions (especially secondary and tertiary cities) are growing much more rapidly than their citizens can be counted - leading to settlements that are, in reality, much larger than their “official” population counts. A good example can be seen in Zinder, Niger, the third largest city in that country: estimated to be growing at more than 5% annually, Zinder has nearly doubled in size since the last official census in 2012, and will likely double again by 2034. Yet, it's difficult to know for sure.
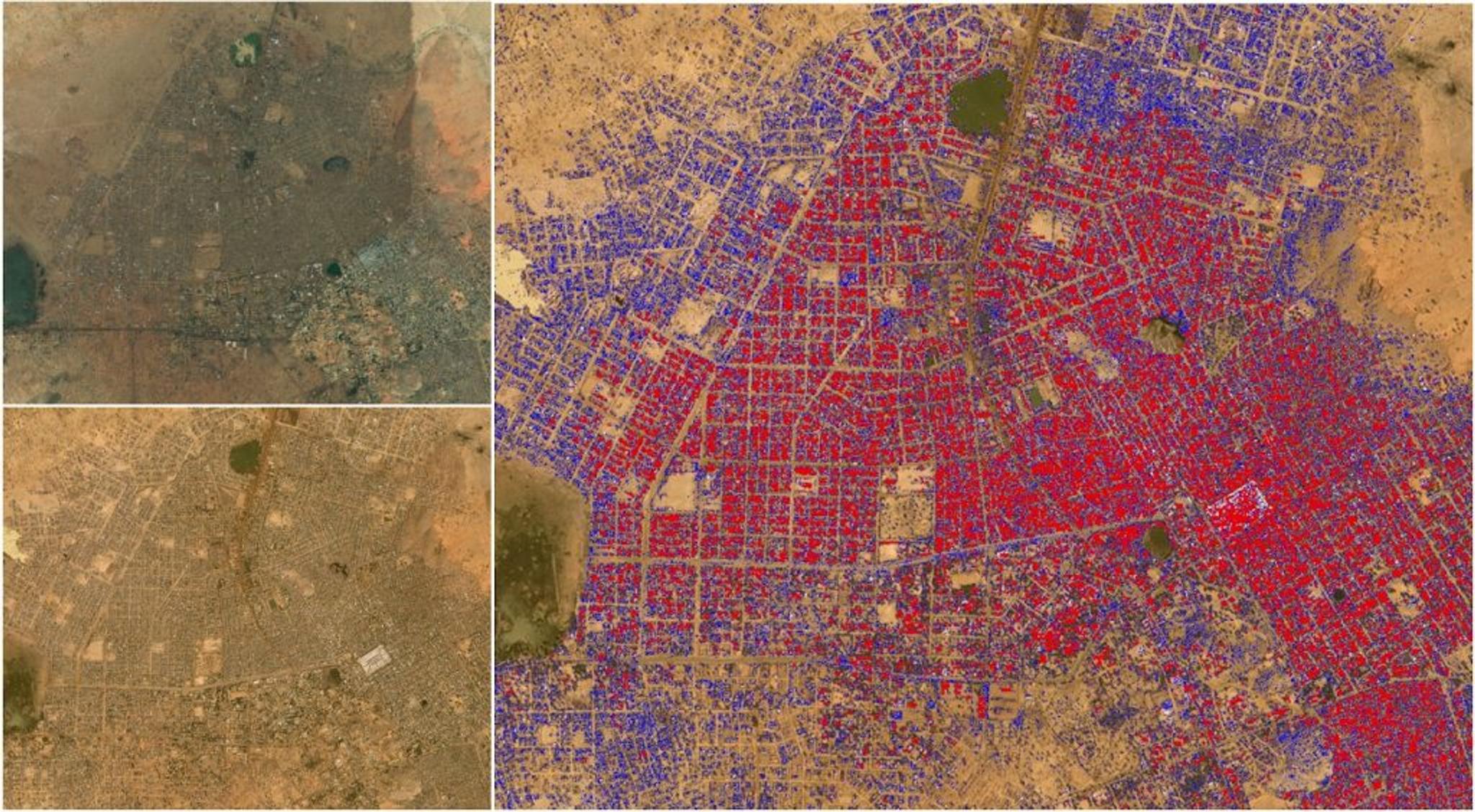
Zinder, Niger, as seen in 2012 in aerial imagery (upper left), and in PlanetScope imagery Q1 2023 (lower left). In the rightmost image, new urban construction (since the 2012 census) is denoted in purple.
Simultaneously, a host of climate-linked risks, including intensifying natural disasters, land-use change and conflict, are driving both voluntary and forced migration. As a result, many population maps, particularly in disruption-prone, low-resource settings, become quickly out of date, effectively rendering some communities invisible. And in an era of serious climate-linked disasters, that invisibility can prove fatal.
Consider the “climate-supercharged” monsoon rains that devastated Pakistan in 2022, leading to torrential flooding in which an estimated 1,700 people lost their lives and nearly 13,000 people were injured. Damage to the food system and mass displacement led, in turn, to chronic food and healthcare shortages that have threatened the growth and development of children in the affected areas, and spurred an increase in malaria cases.
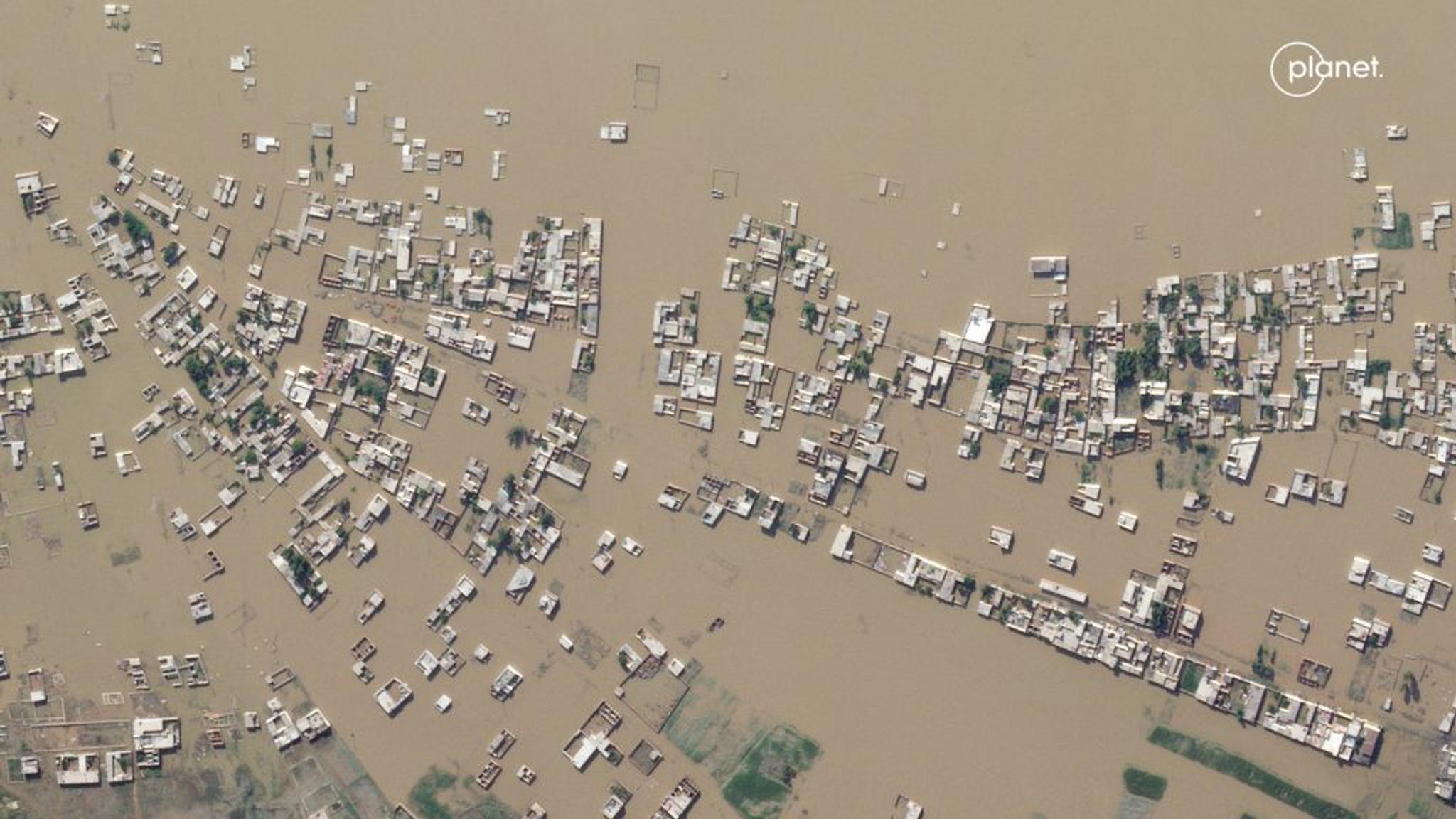
Planet SkySat image of devastating floods in Nowshera, Pakistan.
Climate change intensifies disasters, which drives displacement. That can, among many impacts, push internally displaced people from rural areas into urban slums. That in turn raises the risks of forced evictions and secondary urban displacement, as well as increased risks of public health crises, and so on, in a dispiriting causal chain. Knowing, in detail, where people are located is foundational to breaking that chain, by illuminating what risks communities face; targeting efforts to mitigate those risks as they intensify; and ensuring effective humanitarian action when crises strike.
That’s exactly the aim of a new collaboration between Planet, Microsoft’s AI for Good Lab and the Institute for Health Metrics and Evaluation (IHME) at the University of Washington. Together, we’re using satellite imagery, AI and spatial demography to develop high-resolution, comprehensive, and up-to-date population maps, starting in low-resource/high-climate-stress countries. The resulting maps can be used to help model exposure to climate-linked migration risks, food insecurity, disease burden, and many other contemporary hazards.
The work begins with the collection of comprehensive data from Planet’s Dove satellites, which image the terrestrial land-mass of the Earth nearly every day. This data is assembled into high-quality, high-recency, cloud-free basemaps at 4.7m/pixel resolution, which are then used for subsequent analysis.
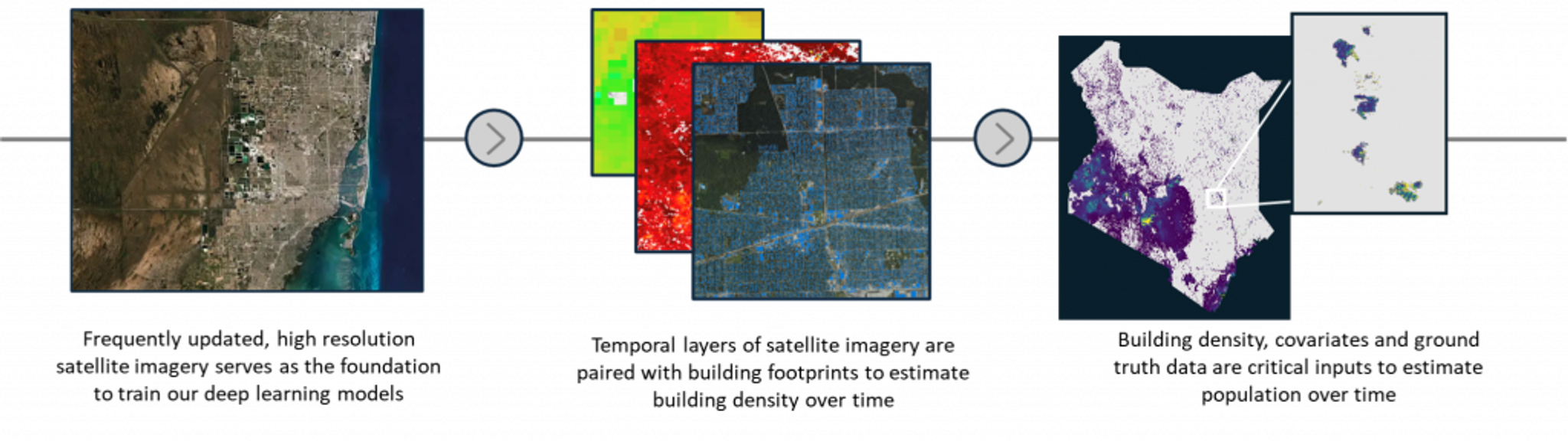
Planet’s high-resolution satellite imagery, the AI for Good Lab’s analytical tools, and IHME’s spatial demographic analysis yield accurate population estimates even in locations with geographic or temporal gaps
Once collected, the team at Microsoft’s AI for Good Lab run machine-learning models on Planet’s quarterly basemaps – 52 trillion pixels for each year of data – to create building density maps, over time, at a ~40m/pixel resolution. The models are trained on samples of precise building footprint labels from across the globe, leveraging work from the SpaceNet7 competition, weak footprint data from the Microsoft building footprint dataset, and hard negative samples from an internal labeling pipeline. The resulting dataset encodes where structures are globally, and how the density of such structures have changed since late 2017, in a way that can easily be used in gridded spatial models.
IHME then uses the building density data as part of its ongoing global modeling efforts to understand health and the determinants of health in every part of the world. This effort includes highly detailed national census data, survey and administrative data, as well as predictors like lights at night, distance from major roadways, fresh water sources, urban/rural classification, and other climate factors.
IHME constructs a geostatistical model of the count of people per human structure. This foundational model allows researchers to predict the distribution of population directly from changes in human structures.
The result is an up-to-date, high-resolution population map that has enormous value for civil government applications, from planning and undertaking a census, estimating budgets for service delivery, citing infrastructure like roads, schools, hospitals and sanitation facilities, and more.
“They also have particular value for public health,” notes IHME’s founder and director Dr. Christopher Murray. “Different diseases move through populations differently depending on how those populations are clustered. By understanding how and where people are located, we can understand risks to populations, and undertake more effective disease surveillance and control strategies.”
The utility of these assets go well beyond measuring disease burden, however. When overlayed with spatial models of climate risks like extreme weather, or food insecurity, they can suggest how many people are subject to various risks - and where. That, in turn, enables anticipatory action – efforts to help exposed populations before a disruption strikes, to prevent or reduce its humanitarian impacts.
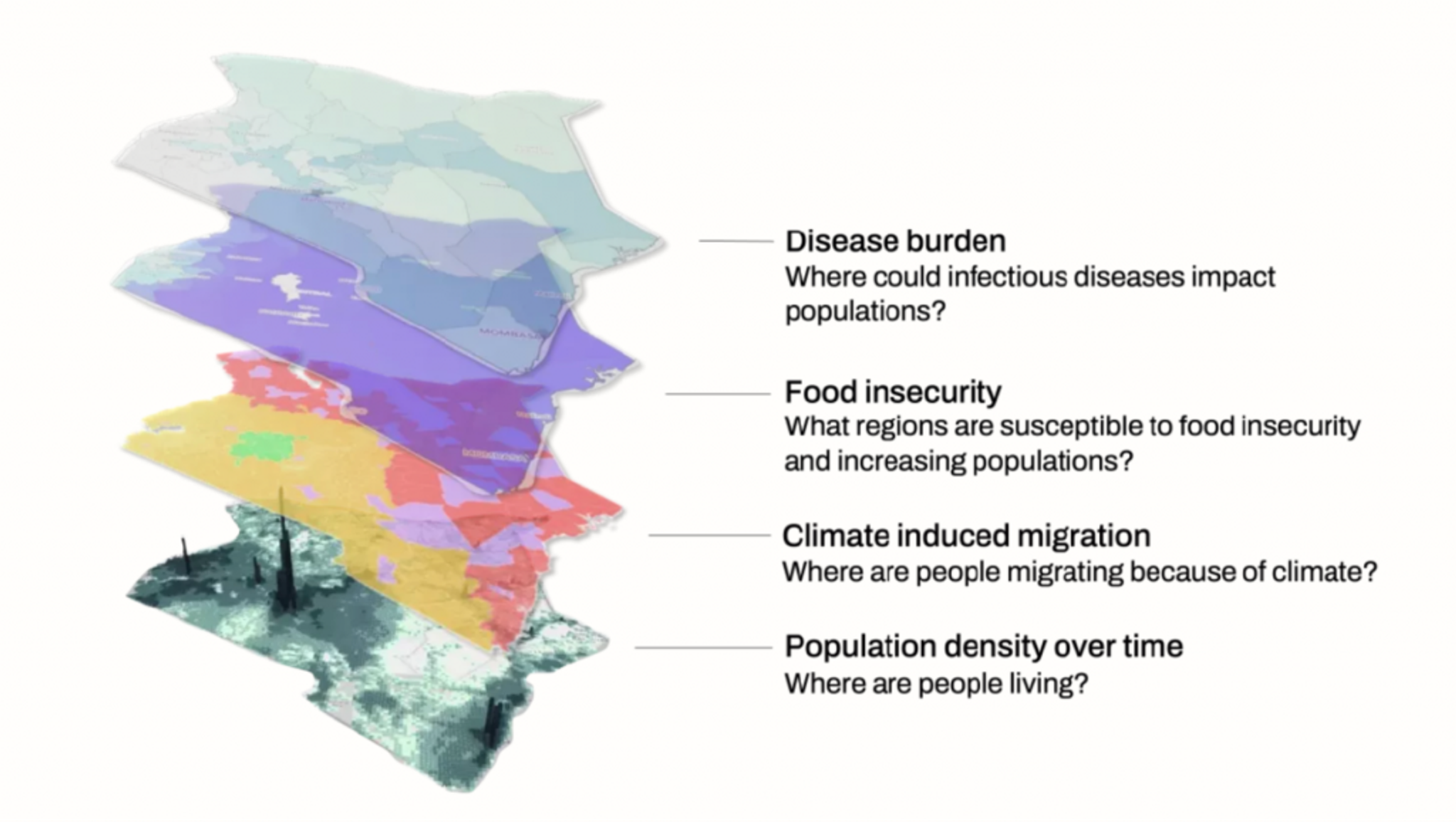
By layering multiple hazards, a composite picture of future risks emerges
“This is exactly the kind of work the AI for Good Lab was established to work on - combining the talents of several world-class partners to do things previously impossible,” said Juan Lavista Ferres, the Lab’s Director and Chief Data Scientist. “In the right hands, we think these assets can help governments and NGOs better serve their citizens at a time when disruptions are expected to become increasingly severe and frequent.”
To learn more about this project, please contact population@planet.com
Catch more on this unique project in this video from The Prompt with Trevor Noah.
Ready to Get Started
Connect with a member of our Sales team. We'll help you find the right products and pricing for your needs