Sensor Fusion of Planet, Landsat and MODIS Data for Unprecedented Land Surface Monitoring
In the midst of a revolution Earth Observation, due to increasingly diverse and temporally dense data feeds enabled by cubesats and other sensors, there is a need to be interoperable across sensors. In the journal Remote Sensing of Environment, Rasmus Houborg and Matt McCabe present the Cubesat-enabled Spatio-Temporal Enhancement Method (CESTEM), which uses multi-scale machine learning to stabilize radiometry across Planet’s flocks of cubesats. By ingesting nearly-coincident images from Planet, Landsat and MODIS with this machine learning technique, Houborg and McCabe are able to produce data feeds consistent with Landsat 8 radiometry, but at the spatial and temporal resolution of Planet’s cubesats (i.e., 3.7m, daily). The authors note that “with the observing potential of Planet’s CubeSats approaching daily nadir-pointing land surface imaging of the entire Earth, CESTEM offers the capacity to produce daily Landsat 8 consistent VNIR imagery with a factor of 10 increase in spatial resolution and with the radiometric quality of actual Landsat 8 observations.” [caption id="attachment_139066" align="aligncenter" width="841"]
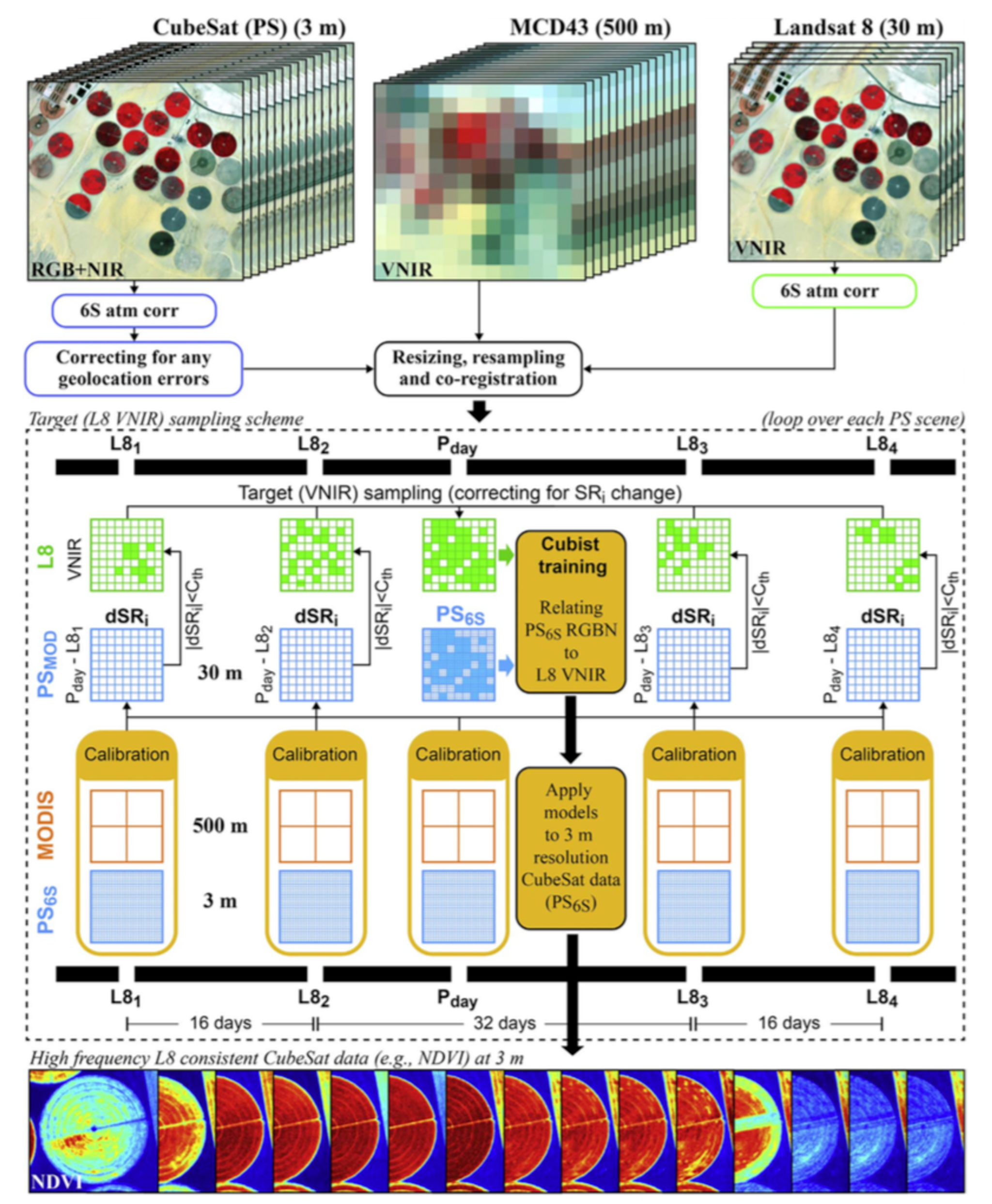
Ready to Get Started
Connect with a member of our Sales team. We'll help you find the right products and pricing for your needs