You Can’t Fix What You Can’t See: The Realities of AI and Satellite Data
Earth observation (EO), the monitoring of the Earth from space using satellites, has undergone fundamental changes in the last decade. We have seen the convergence of two exciting trends in remote sensing and processing algorithms that now herald a new era of space renaissance. The implementation of ambitious government initiatives such as the European Union’s Copernicus Programme, and an explosion in commercial satellite sensing constellations like Planet’s, has been matched by incredible breakthroughs in algorithm performance. This is due to advancements in accelerated computing, open source software, and broadly accessible training data. From a time of relative data paucity, we have entered an era where data exploitation is often compared to taking a drink from a fire hydrant. This change in the availability of what is considered quite high spatial resolution (5-10 m pixels) image data has left many potential users reeling from the opportunities of these new-found riches, and the EO service industry IS scrabbling for the best ways to deal with the data deluge. At the forefront of the strategies to convert vast amounts of data into information has been artificial intelligence (AI), a system able to perform human tasks such as speech recognition, visual perception and decision-making. In the case of vast amounts of image data, AI has the potential to help humans extract insights from huge volumes of unstructured spatiotemporal data and facilitate the process of information discovery. In short, no number of humans would be able to analyze the millions of images being sent down to Earth daily—but, potentially—an AI-capable machine could support this. An obviously powerful tool, AI attracts a great deal of suspicion and criticism and has rather negative connotations for some, such as George Orwell’s Big Brother in the novel 1984, the HAL9000 in 2001: A Space Odyssey, or SkyNet in Terminator. Others see unregulated and unaccountable forms of surveillance supported by AI as a threat to privacy and an intrusion on our lives. A more rational fear is around the uncritical use of AI by computer engineers who have received little training in the issues being addressed and may be unaware of existing solutions which may be relevant. AI could also be an amplifier of the power imbalances between data, information suppliers and users, and the general public. Therefore, can we trust an AI solution to be appropriate and unbiased? [caption id="attachment_143584" align="aligncenter" width="4928"]
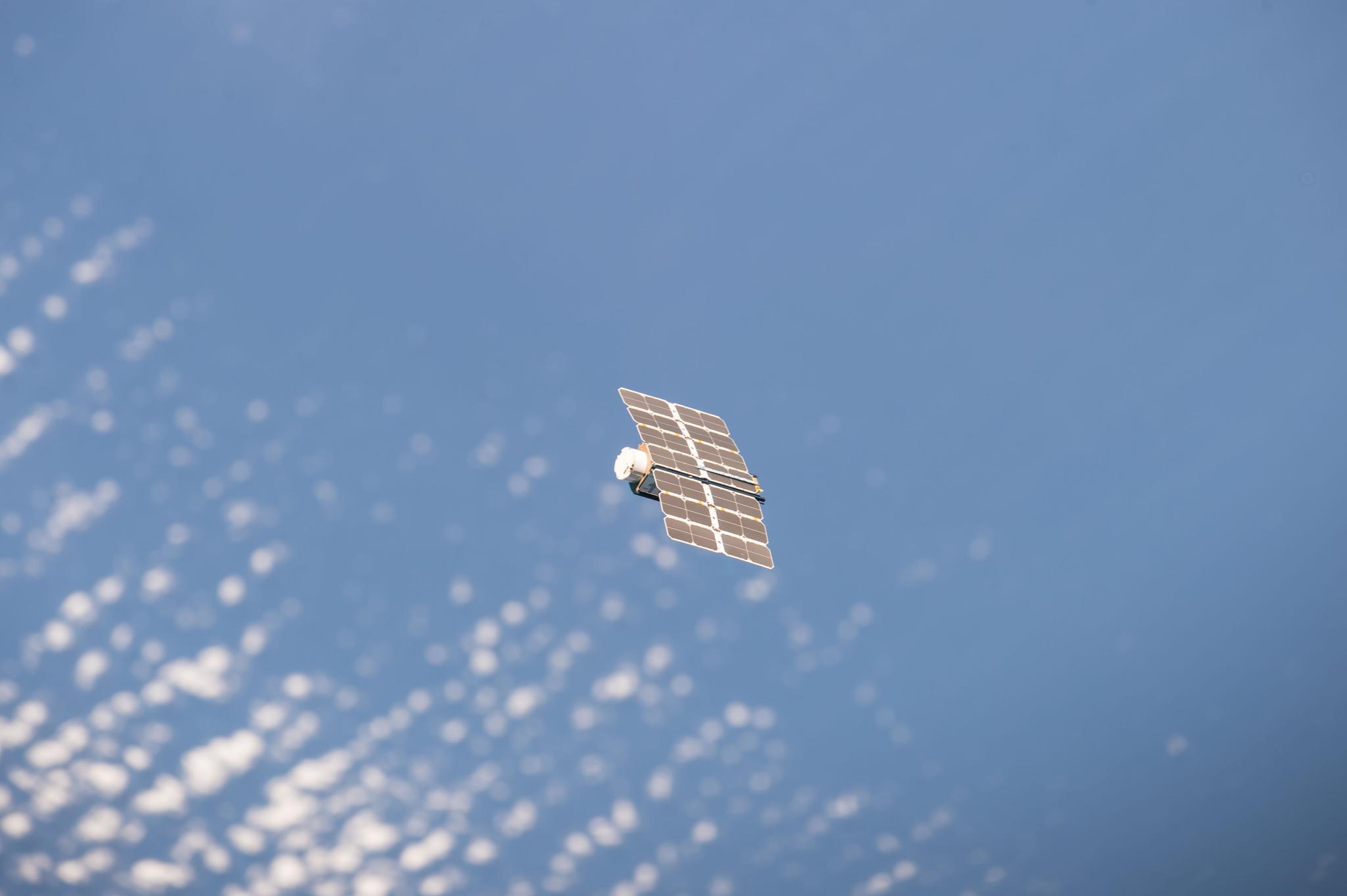
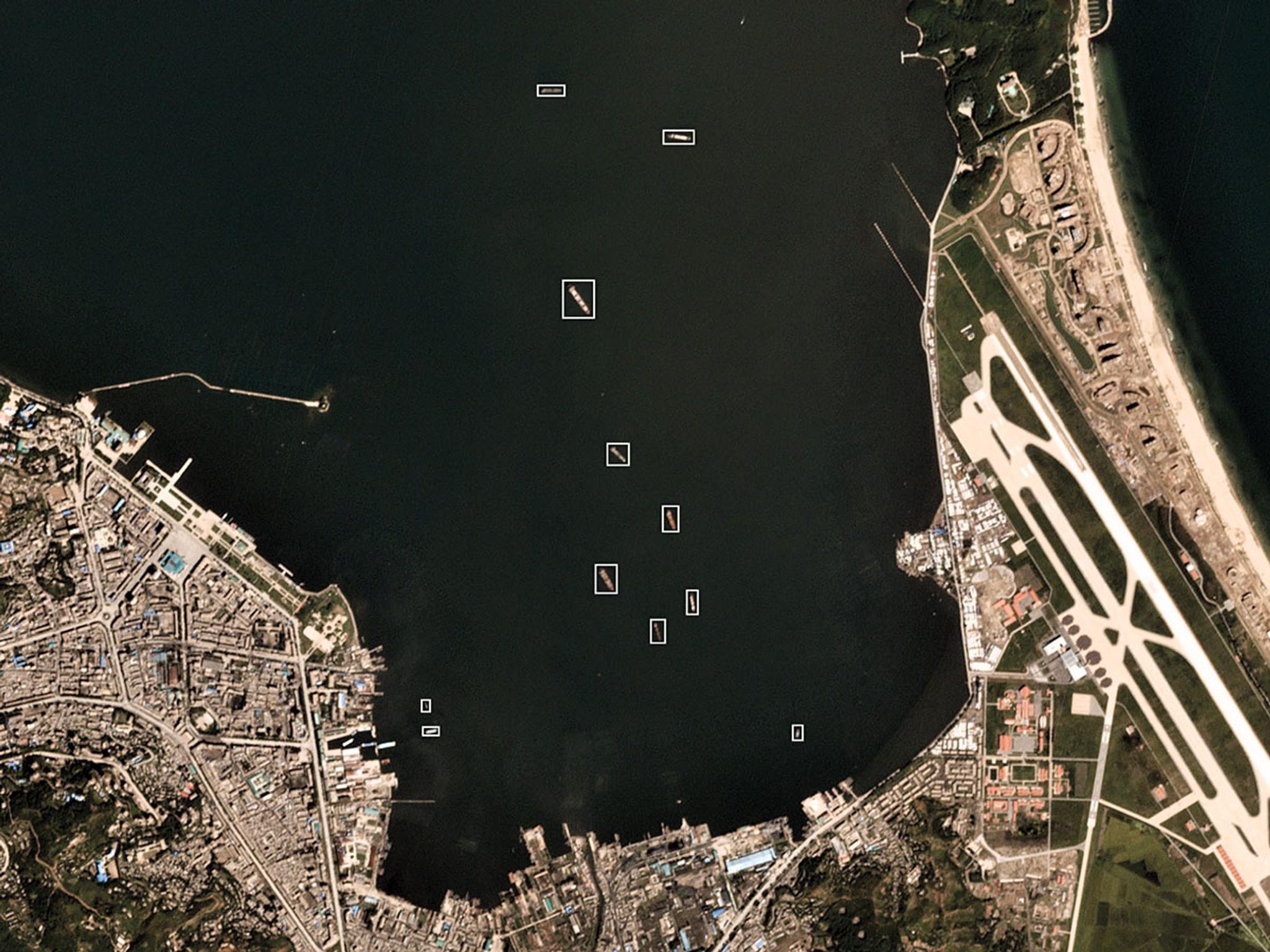
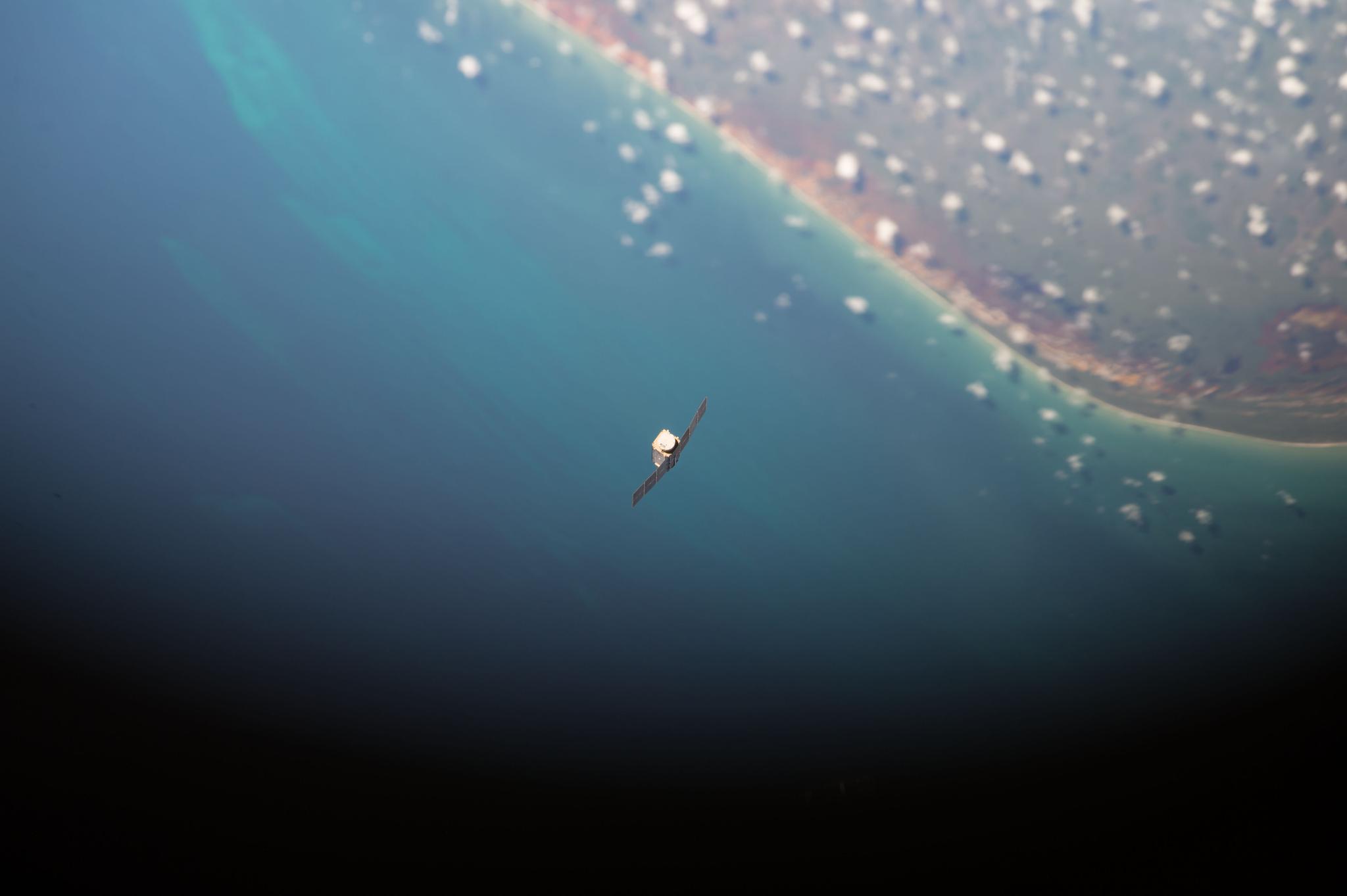
Ready to Get Started
Connect with a member of our Sales team. We'll help you find the right products and pricing for your needs